Fleet Safety Technology: Anomaly Detection in Machine Learning
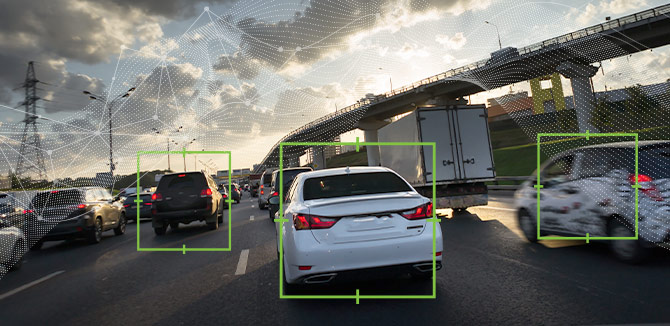
The vast majority of miles driven by the nation’s commercial truck drivers are done safely and without incident. It’s only in those critical moments when the fates converge to create a perfect storm of risky conditions that need to be managed.
The challenge for fleet safety technologies, then, is to help locate those brief, unusual moments and enable drivers and safety managers to steer clear of the dangers. This ability is called anomaly detection, and it’s a powerful form of machine learning that has the potential to significantly improve driver safety, said Stephen Krotosky, manager of applied machine learning at Lytx.
Anomaly Detection in Action
Anomaly detection is already being deployed in the real world. Lytx technology, for example, combines pattern recognition algorithms, computer vision, and machine learning to track patterns of behavior such as lane departure and following distance. BAE Systems, a British defense contractor, uses machine learning algorithms for anomaly detection to sift through fleet and vehicle status data and successfully flag potential maintenance issues overlooked by normal vehicle diagnostics systems. And the National Aeronautics and Space Administration (NASA) uses machine learning to detect anomalies on board its spacecrafts in order to fix mechanical or systems issues before they lead to failure.
“It’s a different approach to finding things,” Krotosky said. “With fleet safety, most of things we look for are anomalous, comparatively rare — collisions, lane departures, cell phone use and other distractions, and so forth. These are relatively rare events in the grander scope of driving.”
Accuracy Matters in Anomaly Detection
That’s why accuracy in anomaly detection is paramount. Let’s say, for example, a system has a false positive rate of one in 1,000. While that sounds low, let’s apply that to the real world where an event is likely to occur one in 1 million times.
The system will ping 100 times in that scenario, but only one of those pings will be accurate. The other 99 are false positives. Think of how often car alarms go off, for example. If false alarms happen too frequently, people stop paying attention, and the alarm ceases to have an effect.
The same can be true of fleet safety systems. This is especially relevant for systems that have in-cab alerts for such events as when the vehicle drifts into another lane, is following another vehicle too closely, or fails to make a complete stop at a stop sign, for example.
“For many applications, the goal is to get false positives below one in 10,000 in the real world,” Krotosky said. “Fully automating this is a challenge.”
Krotosky is up for the challenge. He and his team of researchers are actively developing smarter, more accurate algorithms to help fleets solve a number of business problems — from safety and compliance, to operational efficiency and service optimization.
The World’s Largest Video Telematics Database
It helps that Krotosky has access to a toolbox that contains one of the world’s largest video telematics database of real world commercial driving available, one that has 20 years worth of data from more than 100 billion miles driven by thousands of operators in dozens of vehicle types and in all types of road and weather conditions throughout the 50 states.
That’s like having a virtual professional driver who’s literally seen it all as your primary consultant, helping to make your anomaly detection models smarter and more accurate.
“We’ve seen all kinds of behaviors,” Krotosky said, including reading while driving, among other oddities. “Once we can monitor for a specific behavior, such as cell phone use, we can hopefully work to influence that behavior and reduce risk.”
Learn more about anomaly detection with our DriveCam device.